The brand-new method permits researchers to much better comprehend neural network habits. The neural networks are more difficult to trick thanks to adversarial training.Los Alamos National Laboratory scientists have actually established an unique approach for comparing neural networks that checks out the “black box” of expert system to assist scientists understand neural network habits. Neural networks recognize patterns in datasets and are used in applications as varied as virtual assistants, facial acknowledgment systems, and self-driving automobiles. “The expert system research study neighborhood does not always have a total understanding of what neural networks are doing; they provide us excellent outcomes, however we do not understand how or why,” stated Haydn Jones, a scientist in the Advanced Research in Cyber Systems group at Los Alamos. “Our brand-new approach does a much better task of comparing neural networks, which is a vital action towards much better comprehending the mathematics behind AI.” Scientists at Los Alamos are taking a look at brand-new methods to compare neural networks. This image was produced with an expert system software application called Stable Diffusion, utilizing the timely “Peeking into the black box of neural networks.” Credit: Los Alamos National Laboratory Jones is the lead author of a current paper provided at the Conference on Uncertainty in Artificial Intelligence. The paper is a crucial action in defining the habits of robust neural networks in addition to studying network resemblance. Neural networks are high-performance, however vulnerable. Self-governing automobiles use neural networks to acknowledge indications. They are rather skilled at doing this in ideal situations. The neural network, nevertheless, might incorrectly discover an indication and never ever stop if there is even the tiniest irregularity, like a sticker label on a stop indication. In order to enhance neural networks, scientists are browsing for techniques to increase network toughness. One cutting-edge technique includes “assaulting” networks as they are being trained. The AI is trained to ignore problems that scientists actively present. In essence, this method, called adversarial training, makes it harder to fool the networks. In an unexpected discovery, Jones and his partners from Los Alamos, Jacob Springer and Garrett Kenyon, in addition to Jones’ coach Juston Moore, used their brand-new network resemblance metric to adversarially qualified neural networks. They found that as the intensity of the attack increases, adversarial training triggers neural networks in the computer system vision domain to assemble to really comparable information representations, despite network architecture. “We discovered that when we train neural networks to be robust versus adversarial attacks, they start to do the exact same things,” Jones stated. There has actually been a substantial effort in market and in the scholastic neighborhood looking for the “ideal architecture” for neural networks, however the Los Alamos group’s findings show that the intro of adversarial training narrows this search area considerably. As an outcome, the AI research study neighborhood might not require to invest as much time checking out brand-new architectures, understanding that adversarial training triggers varied architectures to assemble to comparable options. “By discovering that robust neural networks resemble each other, we’re making it simpler to comprehend how robust AI may actually work. We may even be revealing tips regarding how understanding takes place in people and other animals,” Jones stated. Referral: “If You’ve Trained One You’ve Trained Them All: Inter-Architecture Similarity Increases With Robustness” by Haydn T. Jones, Jacob M. Springer, Garrett T. Kenyon and Juston S. Moore, 28 February 2022, Conference on Uncertainty in Artificial Intelligence.
Read More
New Method Exposes How Artificial Intelligence Works
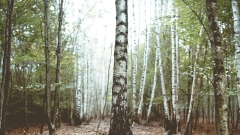